UAV imaging: the future of yield prediction research
The Agricultural University of Athens (AUA) is the third oldest university in Greece. Since 1920, the university has contributed consistently and continuously to Greek and European primary sector development by conducting basic and applied research in agriculturally related sciences. AUA carries out 30% of the agricultural research in Greece.
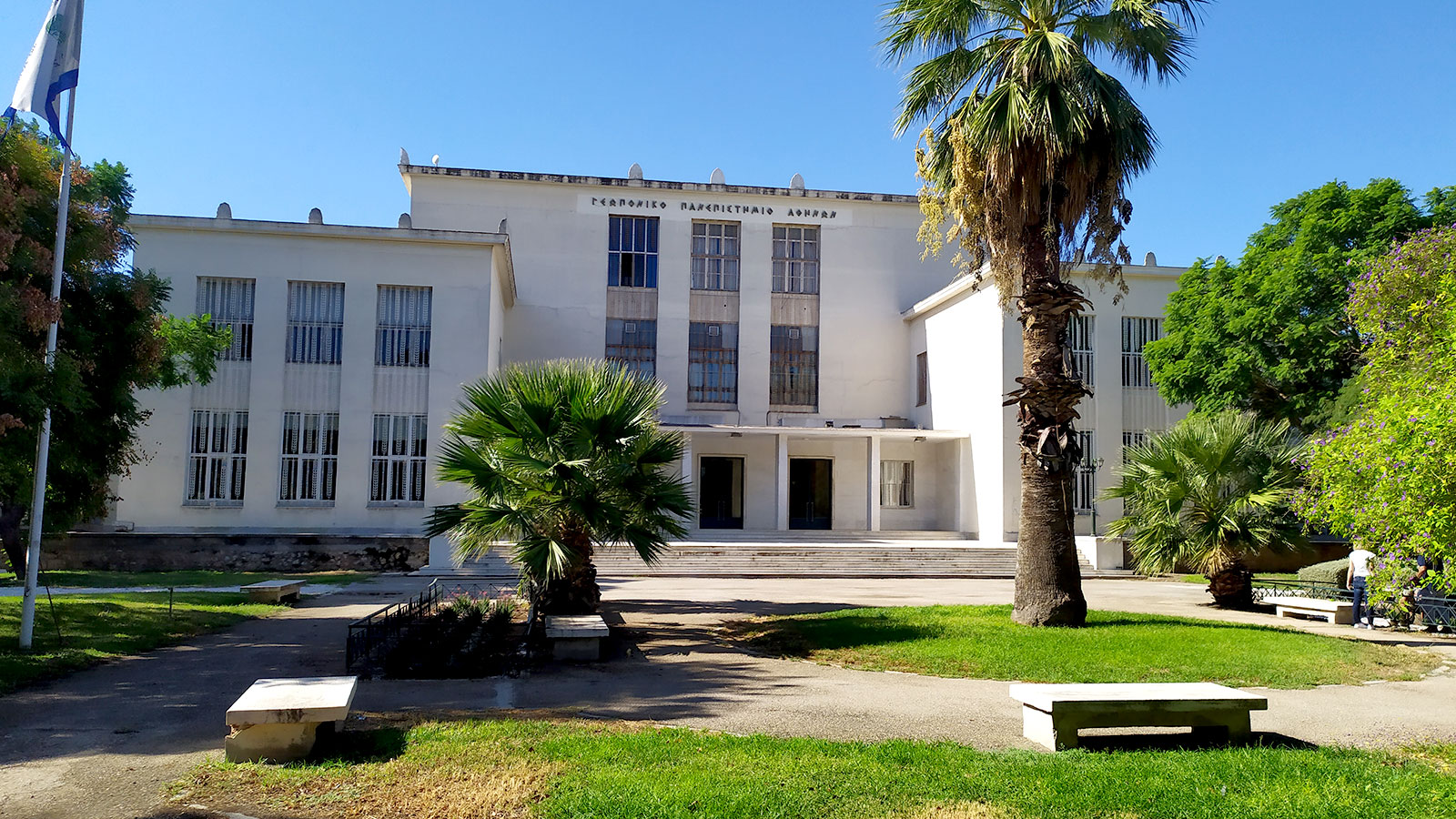
Led by professor Dr. Spyros Fountas, the Smart Farming Technology Group (SFTG) belongs to AUA’s Department of Natural Resource Management and Agricultural Engineering. Among other fields, SFTG members deal with the subjects of metrology in agricultural engineering, automation in agriculture, precision farming, robotics, and AI.
SFTG consists of over 20 research associates and is equipped with multiple types of proximal, multispectral, and hyperspectral sensors and cameras for crop and soil monitoring, several drones equipped with multispectral and thermal cameras along with certified pilots and workload operators, a wide range of commercial state of the art agricultural machinery and robotic systems, as well as high-end PC-s and workstations for deep-learning applications.
Precision agriculture for crop management
The SFTG currently coordinates the H2020 OPTIMA Project for the development of an Optimized Integrated Pest Management system to precisely detect and control plant diseases in perennial crops and open-field vegetables. It is a WP leader in the H2020 project BigDataGrapes, WP leader in H2020 Innoseta thematic network, and has participated in two more H2020 projects in the field of precision agriculture as partners, namely IoF2020 and APOLLO. Additionally, the SFTGroup coordinated the 2020 Smart-AKIS thematic network on innovation-driven research in smart farming technologies and the H2020 GATES project for the development of a serious game for precision agriculture adoption in the EU.
In SmartAgriHubs, under the Flagship Innovation Experiment #26 coordinated by AUA, a yield estimation model for organic open-field broccoli was developed in Marathon, Greece, as one of the 3 key outputs of the Innovation Experiment. The project started in Summer 2019 and is currently in its last month of implementation (ending in June 2021).
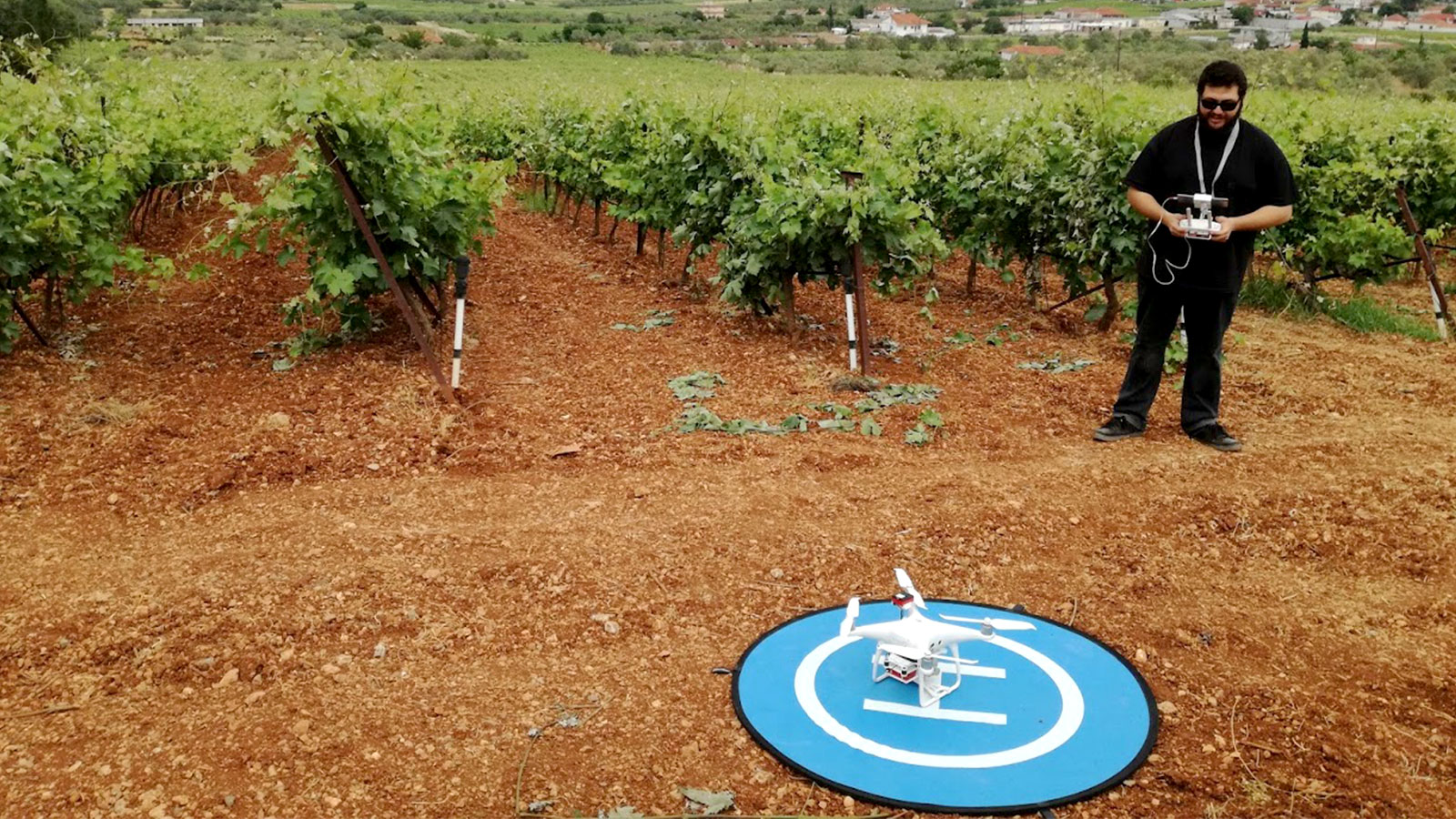
In BigDataGrapes, SFTG collects aerial and proximal crop data from three test sites located in the north-eastern part of Peloponnese, Greece, to denote associations and correlations between precision agriculture information and phenological data. The project took place over 3 years, from 2019 to 2021.
Projects details
Area covered: | Various fields, ranging from 0.25 to 10 ha |
Number of images: | Approx. 70,000-80,000 images for the three projects mentioned here. |
Camera: | Parrot Sequoia |
Drone: | DJI Phantom 4 |
Software | PIX4Dmapper, ArcGIS |
Image resolution: | 1280 x 960 |
GSD: | Depending on the flight high and field size: approx. 1 – 4 cm Smaller fields - 30 m AGL Bigger fields - 70-80 AGL |
Computer specs: | CPUs: Intel I7, various generations (8-9) GPUs: NVIDIA GeForce 1070 Ti, 2080 Ti, 3070 and 3090 |
Processing time: | Depending on the machine used each time and the field / data volume the processing in PIX4Dmapper ranged from 10 to 30 minutes |
In a research project funded by Corteva Agriscience Hellas, 13 tomato processing producers participated in the project, with 3 tomato hybrids. 'Processing tomatoes' is a term for the tomato varieties that are cultivated in open fields, getting its name from the fact that they are meant to produce "processed" tomato products, such as canned tomato pulp or ketchup. SFTG collected UAV data from all fields throughout the growing season and studied the correlations between each measurement and the recorded yield from the fields during harvesting to develop a yield estimation model for processing tomatoes. The project started in 2019 and is currently at the beginning of its 3rd year of implementation which will take place this summer (2021).
Crop yield is the most important piece of information for crop management in precision agriculture but very few crop-specific tools have been developed. Early crop yield estimations allow farmers to optimize farm operation schedules, field management, and product marketing decisions. The outputs of the project provide valuable tools for the farmers, allowing them to estimate the expected income of each production unit, while also enabling the efficient transfer of the production from the farm to the food supply chain.
PIX4Dmapper for yield estimation
The AUA team used PIX4Dmapper Ag Multispectral processing template to generate georeferenced orthomosaic maps of our fields and extract the monochromatic TIF raster files. They additionally used ArcGIS to perform geostatistical analysis, such as zonal statistics for their experimental grids.
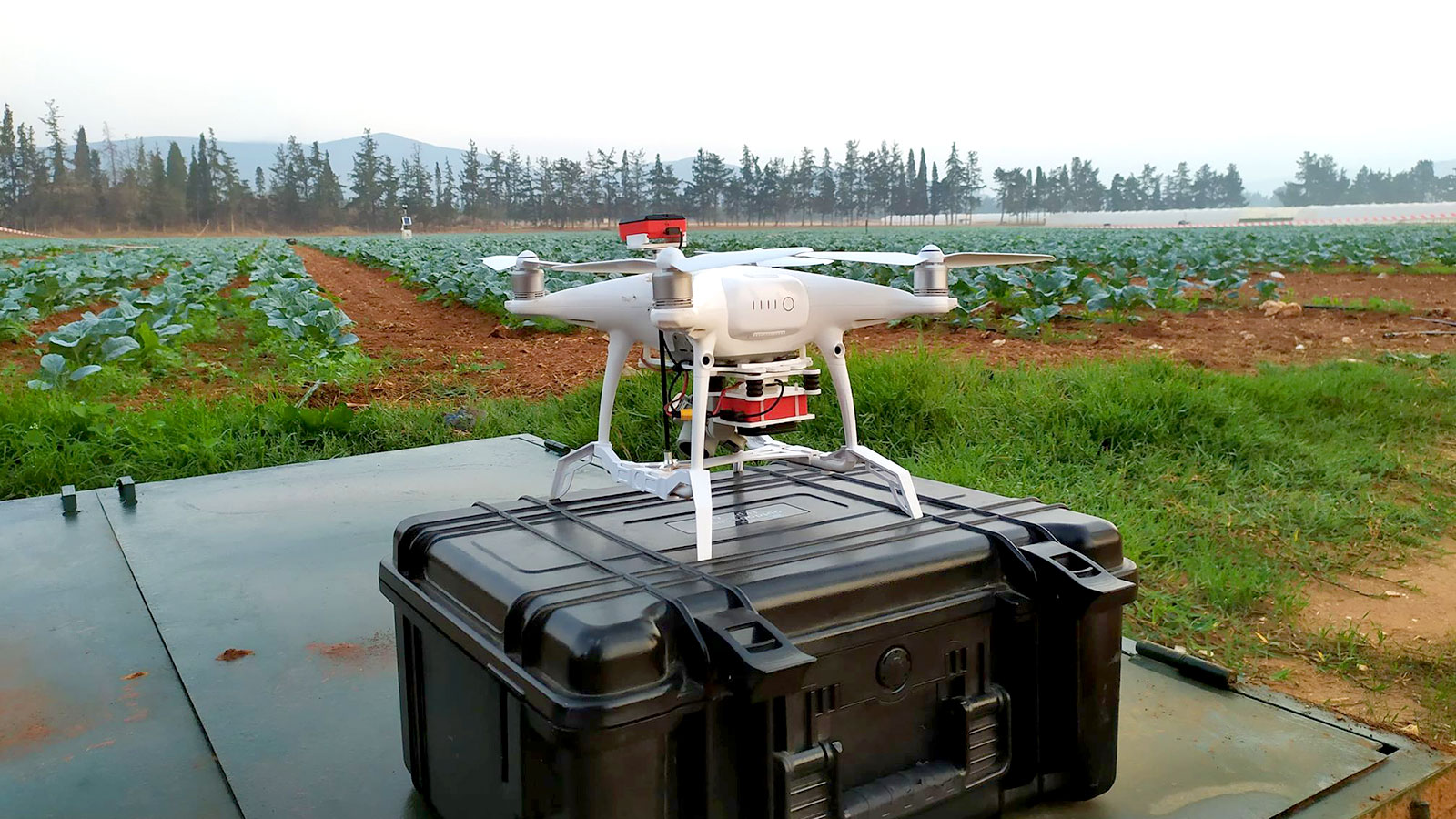
Depending on the field size, the AUA team flew at lower altitudes (30m AGL) for smaller fields and flew higher (70-80m AGL) for larger ones. This resulted in their GSD ranging from approximately 1 to 4cm. The GPS accuracy of their UAVs ranges from 20cm for custom drones to approx. 1m for simple commercial ones.
"We consider Pix4D to be the ideal tool for cases where a large number of flights are processed within the same team/group, because of its efficiency and ease of use of various ready-to-use models and pipelines. Additionally, its user-friendly interface makes it ideal for students that just started working with UAV imagery."
Vasilis Psiroukis, Agricultural Engineer and AUA Research Associate
“The main reason that we selected Pix4D as our UAV photogrammetric software is that it offered a very reliable and consistent photogrammetry tool, with a very easy implementation and user-friendly interface, as well as its compatibility with Parrot Sequoia, one of the most widely used UAV multispectral sensors across the world”, points out Vasilis Psiroukis, Agricultural Engineer and AUA Research Associate.
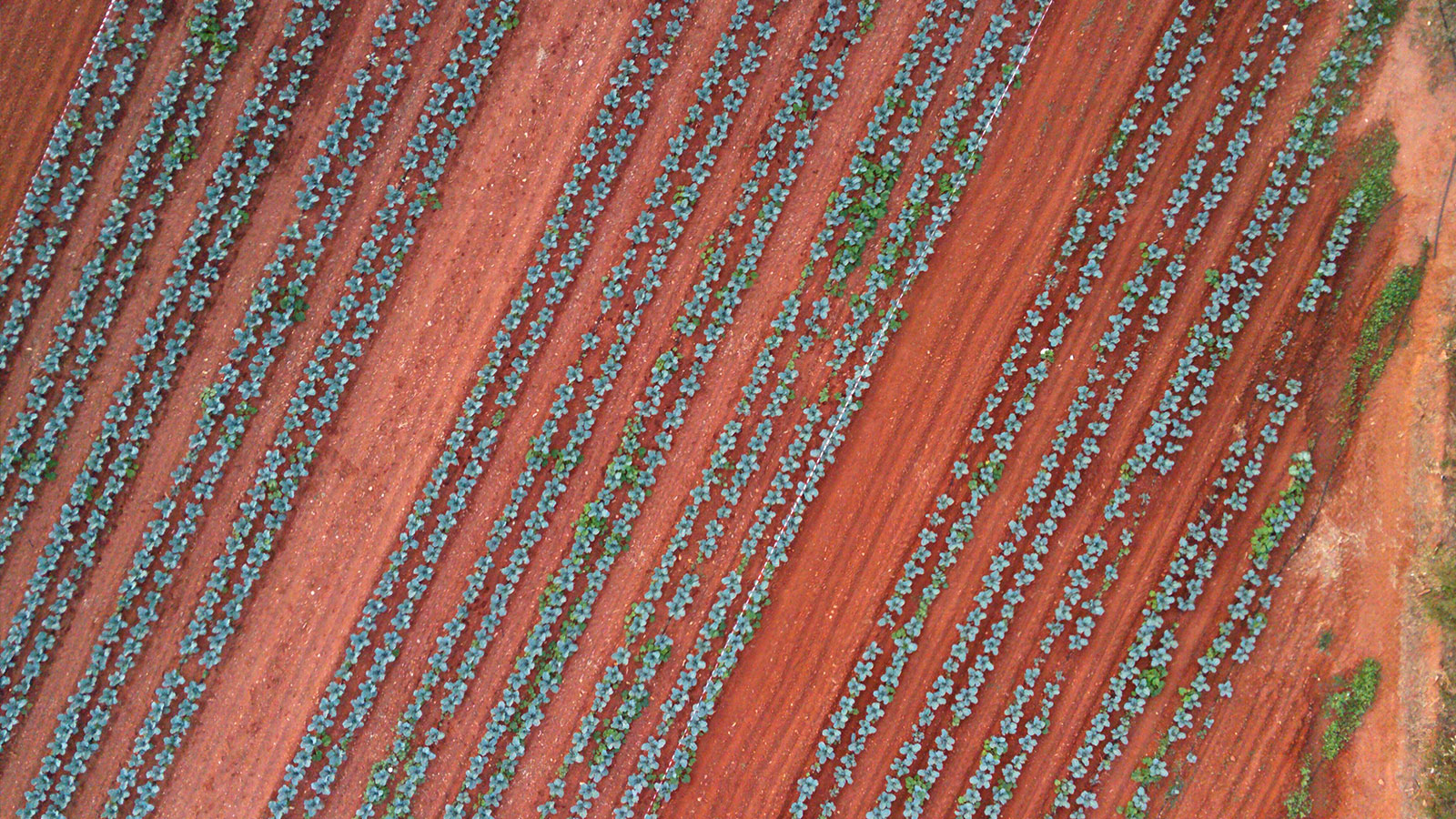
The future of yield prediction research
Outputs from these 3 projects, in a yield estimation context, provide tools for local and European farmers that allow them to estimate their production weeks ahead so that they can better manage harvesting operations and agrifood chain logistics.
Yield estimation is an extremely important piece of information when becoming accessible in a timely manner. The data used, however, should be of the highest quality; otherwise, the modeling process can become prone to error. All field measurements require a significant amount of time and effort to generate high quality data. However, UAVs have managed to solve this problem, and this is why they believe that UAV imaging is the future of yield prediction research.